Deep Learning. A Practitioner's Approach - Helion
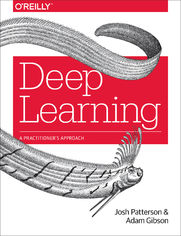
ISBN: 978-14-919-1421-2
stron: 532, Format: ebook
Data wydania: 2017-07-28
Księgarnia: Helion
Cena książki: 186,15 zł (poprzednio: 216,45 zł)
Oszczędzasz: 14% (-30,30 zł)
Although interest in machine learning has reached a high point, lofty expectations often scuttle projects before they get very far. How can machine learning—especially deep neural networks—make a real difference in your organization? This hands-on guide not only provides the most practical information available on the subject, but also helps you get started building efficient deep learning networks.
Authors Adam Gibson and Josh Patterson provide theory on deep learning before introducing their open-source Deeplearning4j (DL4J) library for developing production-class workflows. Through real-world examples, you’ll learn methods and strategies for training deep network architectures and running deep learning workflows on Spark and Hadoop with DL4J.
- Dive into machine learning concepts in general, as well as deep learning in particular
- Understand how deep networks evolved from neural network fundamentals
- Explore the major deep network architectures, including Convolutional and Recurrent
- Learn how to map specific deep networks to the right problem
- Walk through the fundamentals of tuning general neural networks and specific deep network architectures
- Use vectorization techniques for different data types with DataVec, DL4J’s workflow tool
- Learn how to use DL4J natively on Spark and Hadoop
Osoby które kupowały "Deep Learning. A Practitioner's Approach", wybierały także:
- Windows Media Center. Domowe centrum rozrywki 66,67 zł, (8,00 zł -88%)
- Ruby on Rails. Ćwiczenia 18,75 zł, (3,00 zł -84%)
- Przywództwo w świecie VUCA. Jak być skutecznym liderem w niepewnym środowisku 58,64 zł, (12,90 zł -78%)
- Scrum. O zwinnym zarządzaniu projektami. Wydanie II rozszerzone 58,64 zł, (12,90 zł -78%)
- Od hierarchii do turkusu, czyli jak zarządzać w XXI wieku 58,64 zł, (12,90 zł -78%)
Spis treści
Deep Learning. A Practitioner's Approach eBook -- spis treści
- Preface
- Whats in This Book?
- Who Is The Practitioner?
- Who Should Read This Book?
- The Enterprise Machine Learning Practitioner
- The practicing data scientist
- The Java engineer
- The Enterprise Executive
- The Academic
- The Enterprise Machine Learning Practitioner
- Conventions Used in This Book
- Using Code Examples
- Administrative Notes
- OReilly Safari
- How to Contact Us
- Acknowledgments
- Josh
- Adam
- 1. A Review of Machine Learning
- The Learning Machines
- How Can Machines Learn?
- Biological Inspiration
- What Is Deep Learning?
- Going Down the Rabbit Hole
- Framing the Questions
- The Math Behind Machine Learning: Linear Algebra
- Scalars
- Vectors
- Matrices
- Tensors
- Hyperplanes
- Relevant Mathematical Operations
- Dot product
- Element-wise product
- Outer product
- Converting Data Into Vectors
- Solving Systems of Equations
- Methods for solving systems of linear equations
- Iterative methods
- Iterative methods and linear algebra
- The Math Behind Machine Learning:Â Statistics
- Probability
- Conditional Probabilities
- Posterior Probability
- Distributions
- Samples Versus Population
- Resampling Methods
- Selection Bias
- Likelihood
- How Does Machine Learning Work?
- Regression
- Setting up the model
- Visualizing linear regression
- Relating the linear regression model
- Classification
- Clustering
- Underfitting and Overfitting
- Optimization
- Convex Optimization
- Gradient Descent
- Stochastic Gradient Descent
- Mini-batch training and SGD
- Quasi-Newton Optimization Methods
- Generative Versus Discriminative Models
- Regression
- Logistic Regression
- The Logistic Function
- Understanding Logistic Regression Output
- Evaluating Models
- The Confusion Matrix
- Sensitivity versus specificity
- Accuracy
- Precision
- Recall
- F1
- Context and interpreting scores
- The Confusion Matrix
- Building an Understanding of Machine Learning
- The Learning Machines
- 2. Foundations of Neural Networks and Deep Learning
- Neural Networks
- The Biological Neuron
- Synapses
- Dendrites
- Axons
- Information flow across the biological neuron
- From biological to artificial
- The Perceptron
- History of the perceptron
- Definition of the perceptron
- The perceptron learning algorithm
- Limitations of the early perceptron
- Multilayer Feed-Forward Networks
- Evolution of the artificial neuron
- Artificial neuron input
- Connection weights
- Biases
- Activation functions
- Comparing the biological neuron and the artificial neuron
- Feed-forward neural network architecture
- Input layer
- Hidden layer
- Output layer
- Connections between layers
- Evolution of the artificial neuron
- The Biological Neuron
- Training Neural Networks
- Backpropagation Learning
- Algorithm intuition
- A closer look at backpropagation
- Understanding backpropagation pseudocode
- Updating the output layer weights
- Further expressing the error term
- The new propagation rule for the error value
- Updating the hidden layers
- Backpropagation Learning
- Activation Functions
- Linear
- Sigmoid
- Tanh
- Hard Tanh
- Softmax
- Rectified Linear
- Leaky ReLU
- Softplus
- Loss Functions
- Loss Function Notation
- Loss Functions for Regression
- Mean squared error loss
- Other loss functions for regression
- Mean absolute error loss
- Mean squared log error loss
- Mean absolute percentage error loss
- Regression loss function discussion
- Loss Functions for Classification
- Hinge loss
- Logistic loss
- Negative log likelihood
- Loss Functions for Reconstruction
- Hyperparameters
- Learning Rate
- Regularization
- Momentum
- Sparsity
- Neural Networks
- 3. Fundamentals of Deep Networks
- Defining Deep Learning
- What Is Deep Learning?
- Defining deep networks
- Evolutionary progress and resurgence
- Advances in network architecture
- Advances in layer types
- Advances in neuron types
- Hybrid architectures
- From feature engineering to automated feature learning
- Feature engineering
- Feature learning
- Generative modeling
- Inceptionism
- Modeling artistic style
- GANs
- Recurrent Neural Networks
- The Tao of deep learning
- Organization of This Chapter
- What Is Deep Learning?
- Common Architectural Principles of Deep Networks
- Parameters
- Layers
- Activation Functions
- Activation functions for general architecture
- Hidden layer activation functions
- Output layer for regression
- Output layer for binary classification
- Output layer for multiclass classification
- Activation functions for general architecture
- Loss Functions
- Reconstruction cross-entropy
- Optimization Algorithms
- First-order methods
- Second-order methods
- L-BFGS
- Conjugate gradient
- Hessian-free
- Hyperparameters
- Layer size
- Magnitude hyperparameters
- Learning rate
- Nesterovs momentum
- AdaGrad
- RMSProp
- AdaDelta
- ADAM
- Regularization
- Dropout
- DropConnect
- L1
- L2
- Mini-batching
- Summary
- Building Blocks of Deep Networks
- RBMs
- Network layout
- Visible and hidden layers
- Â Connections and weights
- Biases
- Training
- Reconstruction
- Other uses of RBMs
- Network layout
- Autoencoders
- Similarities to multilayer perceptrons
- Defining features of autoencoders
- Unsupervised learning of unlabeled data
- Learning to reproduce the input data
- Training autoencoders
- Common variants of autoencoders
- Compression autoencoders
- Denoising autoencoders
- Applications of autoencoders
- Variational Autoencoders
- RBMs
- Defining Deep Learning
- 4. Major Architectures of Deep Networks
- Unsupervised Pretrained Networks
- Deep Belief Networks
- Feature Extraction with RBM Layers
- Learning higher-order features automatically
- Initializing the feed-forward network
- Fine-tuning a DBN with a feed-forward multilayer neural network
- Gentle backpropagation
- The output layer
- Current state of DBNs
- Feature Extraction with RBM Layers
- Generative Adversarial Networks
- Training generative models, unsupervised learning, and GANs
- The discriminator network
- The generative network
- Building generative models and Deep Convolutional Generative Adversarial Networks
- Conditional GANs
- Comparing GANs and variational autoencoders
- Training generative models, unsupervised learning, and GANs
- Deep Belief Networks
- Convolutional Neural Networks (CNNs)
- Biological Inspiration
- Intuition
- CNN Architecture Overview
- Neuron spatial arrangements
- Evolution of the connections between layers
- Input Layers
- Convolutional Layers
- Convolution
- Filters
- Activation maps
- Parameter sharing
- Learned filters and renders
- ReLU activation functions as layers
- Convolutional layer hyperparameters
- Filter size
- Output depth
- Stride
- Zero-padding
- Batch normalization and layers
- Pooling Layers
- Fully Connected Layers
- Other Applications of CNNs
- CNNs of Note
- Summary
- Recurrent Neural Networks
- Modeling the Time Dimension
- Lost in time
- Temporal feedback and loops in connections
- Sequences and time-series data
- Understanding model input and output
- 3D Volumetric Input
- Uneven time-series and masking
- Why Not Markov Models?
- General Recurrent Neural Network Architecture
- Recurrent Neural Networks architecture and time-steps
- LSTM Networks
- Properties of LSTM networks
- LSTM network architecture
- LSTM units
- LSTM layers
- Training
- BPTT and truncated BPTT
- Domain-Specific Applications and Blended Networks
- Modeling the Time Dimension
- Recursive Neural Networks
- Network Architecture
- Varieties of Recursive Neural Networks
- Applications of Recursive Neural Networks
- Summary and Discussion
- Will Deep Learning Make Other Algorithms Obsolete?
- Different Problems Have Different Best Methods
- When Do I Need Deep Learning?
- When to use deep learning
- When to stick with traditional machine learning
- Unsupervised Pretrained Networks
- 5. Building Deep Networks
- Matching Deep Networks to the Right Problem
- Columnar Data and Multilayer Perceptrons
- Images and Convolutional Neural Networks
- Time-series Sequences and Recurrent Neural Networks
- Using Hybrid Networks
- The DL4J Suite of Tools
- Vectorization and DataVec
- Runtimes and ND4J
- ND4J and the need for speed
- JavaCPP
- CPU backends
- GPU backends
- Benchmarking ND4J and DL4J
- ND4J and the need for speed
- Basic Concepts of the DL4J API
- Loading and Saving Models
- Writing a trained model to disk
- Writing to HDFS
- Reading a saved model from disk
- Reading from HDFS
- Writing a trained model to disk
- Getting Input for the Model
- Loading data during training
- Setting Up Model Architecture
- Building layer-oriented architectures
- Hyperparameters
- Training and Evaluation
- Making a prediction
- Training, validation, and test data
- Loading and Saving Models
- Modeling CSV Data with Multilayer Perceptron Networks
- Setting Up Input Data
- Determining Network Architecture
- General hyperparameters
- First hidden layer
- Output layer for classification
- Training the Model
- Evaluating the Model
- Modeling Handwritten Images Using CNNs
- Java Code Listing for the LeNet CNN
- Loading and Vectorizing the Input Images
- Network Architecture for LeNet in DL4J
- General hyperparameters
- Convolution layers
- Max-pooling layers
- Output layer
- Training the CNN
- Modeling Sequence Data by Using Recurrent Neural Networks
- Generating Shakespeare via LSTMs
- High-level modeling workflow
- Java code for modeling Shakespeare
- Setting up input data and vectorization
- LSTM network architecture
- General comments on hyperparameters
- Training the LSTM network
- Generating Shakespeare samples
- Classifying Sensor Time-series Sequences Using LSTMs
- Java code listing for recurrent classification example
- Setting up input data and vectorization
- Network architecture and training
- Generating Shakespeare via LSTMs
- Using Autoencoders for Anomaly Detection
- Java Code Listing for Autoencoder Example
- Setting Up Input Data
- Autoencoder Network Architecture and Training
- Evaluating the Model
- Using Variational Autoencoders to Reconstruct MNIST Digits
- Code Listing to Reconstruct MNIST Digits
- Examining the VAE Model
- Understanding the scatterplot
- Understanding the generated images
- Applications of Deep Learning in Natural Language Processing
- Learning Word Embedding Using Word2Vec
- The Word2Vec model and algorithm
- Modeling context
- Learning similar meaning and semantic relationships
- Vector arithmetic and word embedding
- Java code listing for Word2Vec example
- Understanding the Word2Vec example
- Other practical uses of Word2Vec
- Distributed Representations of Sentences with Paragraph Vectors
- Building paragraph vectors
- Understanding the paragraph vectors example
- Using Paragraph Vectors for Document Classification
- Understanding the paragraph vectors classification example
- Further exploration of the Word2Vec approach
- Extensions into specific domains: Gov2Vec
- Graphs and Node2Vec
- Recommendation engines and Item2Vec
- Computer vision and FaceNet
- Learning Word Embedding Using Word2Vec
- Matching Deep Networks to the Right Problem
- 6. Tuning Deep Networks
- Basic Concepts in Tuning Deep Networks
- An Intuition for Building Deep Networks
- Building the Intuition as a Step-by-Step Process
- Matching Input Data and Network Architectures
- Summary
- Relating Model Goal and Output Layers
- Regression Model Output Layer
- Classification Model Output Layer
- Single-label classification models
- Models with more than two labels
- Multiclass classification models
- Multilabel classification models
- Working with Layer Count, Parameter Count, and Memory
- Feed-Forward Multilayer Neural Networks
- Determining hidden-layer count
- Determining neuron count per layer
- Controlling Layer and Parameter Counts
- Getting the parameter count for a network
- Estimating Network Memory Requirements
- Feed-Forward Multilayer Neural Networks
- Weight Initialization Strategies
- Using Activation Functions
- Summary Table for Activation Functions
- Applying Loss Functions
- Understanding Learning Rates
- Using the Ratio of Updates-to-Parameters
- Specific Recommendations for Learning Rates
- How Sparsity Affects Learning
- Applying Methods of Optimization
- SGD Best Practices
- Using Parallelization and GPUs for Faster Training
- Online Learning and Parallel Iterative Algorithms
- Task parallelism
- Data parallelism
- Parallelizing SGD in DL4J
- Parallel SGD execution
- GPUs
- Online Learning and Parallel Iterative Algorithms
- Controlling Epochs and Mini-Batch Size
- Understanding Mini-Batch Size Trade-Offs
- How to Use Regularization
- Priors as Regularizers
- Max-Norm Regularization
- Dropout
- Issues with dropout
- Other Regularization Topics
- Working with Class Imbalance
- Methods for Sampling Classes
- Weighted Loss Functions
- Dealing with Overfitting
- Using Network Statistics from the Tuning UI
- Detecting Poor Weight Initialization
- Detecting Nonshuffled Data
- Detecting Issues with Regularization
- Basic Concepts in Tuning Deep Networks
- 7. Tuning Specific Deep Network Architectures
- Convolutional Neural Networks (CNNs)
- Common Convolutional Architectural Patterns
- Configuring Convolutional Layers
- Setting the stride for filters
- Using padding
- Choosing the number of filters
- Configuring filter size
- Convolution mode and calculating spatial size of output volume
- Configuring Pooling Layers
- Transfer Learning
- An alternative to training from scratch
- When to consider trying transfer learning
- Recurrent Neural Networks
- Network Input Data and Input Layers
- Output Layers and RnnOutputLayer
- Training the Network
- Initializing weights
- Backpropagation through time
- Regularization
- Debugging Common Issues with LSTMs
- Padding and Masking
- Applying padding and masking to volumetric input
- Evaluation and Scoring With Masking
- Classification using the evaluation class
- Scoring new data with MultiLayerNetwork
- Variants of Recurrent Network Architectures
- Restricted Boltzmann Machines
- Hidden Units and Modeling Available Information
- Using Different Units
- Using Regularization with RBMs
- DBNs
- Using Momentum
- Using Regularization
- Dropout
- Determining Hidden Unit Count
- Convolutional Neural Networks (CNNs)
- 8. Vectorization
- Introduction to Vectorization in Machine Learning
- Why Do We Need to Vectorize Data?
- Strategies for Dealing with Columnar Raw Data Attributes
- Nominal
- Ordinal
- Interval
- Ratio
- Feature Engineering and Normalization Techniques
- Feature copying
- Normalization
- Standardization and zero mean, unit variance
- Min-max scaling
- Whitening and principal component analysis
- Applying normalization in Recurrent Neural Networks and CNNs
- Normalization for regression models
- Binarization
- Using DataVec for ETL and Vectorization
- Vectorizing Image Data
- Image Data Representation in DL4J
- Image Data and Vector Normalization with DataVec
- Working with Sequential Data in Vectorization
- Major Variations of Sequential Data Sources
- Vectorizing Sequential Data with DataVec
- Converting time-series to a single vector
- Converting sequential data to a DataSet object in local mode
- Building custom DataSets from sequential data
- Working with Text in Vectorization
- Bag of Words
- TF-IDF
- TF
- IDF
- Computing the full TF-IDF score
- Comparing Word2Vec and VSM Comparison
- Working with Graphs
- Introduction to Vectorization in Machine Learning
- 9. Using Deep Learning and DL4J on Spark
- Introduction to Using DL4J with Spark and Hadoop
- Operating Spark from the Command Line
- spark-submit
- Working with Hadoop security and Kerberos
- Uploading the Spark assembly
- Initializing Kerberos
- Operating Spark from the Command Line
- Configuring and Tuning Spark Execution
- Running Spark on Mesos
- Running Spark on YARN
- Comparing Spark execution modes
- General Spark Tuning Guide
- Setting the number of executors
- Spark executors and CPU cores
- Spark executors and memory
- Spark and YARN container resource allocation
- Understanding executor memory requests in YARN
- Understanding Spark, the JVM, and garbage collection
- Dealing with slowing garbage collection efficiency or pauses
- Selecting a garbage collector for the JVM and Spark
- Tuning DL4J Jobs on Spark
- Tuning the number of executors
- Tuning the amount of memory for executors
- Setting Up a Maven Project Object Model for Spark and DL4J
- A pom.xml File Dependency Template
- Setting Up a POM File for CDH 5.X
- Setting Up a POM File for HDP 2.4
- Troubleshooting Spark and Hadoop
- Common Issues with ND4J
- ND4J and Kyro serialization
- jnind4j and java.library.path
- Common Issues with ND4J
- DL4J Parallel Execution on Spark
- A Minimal Spark Training Example
- DL4J API Best Practices for Spark
- Multilayer Perceptron Spark Example
- Setting Up MLP Network Architecture for Spark
- Distributed Training and Model Evaluation
- Building and Executing a DL4J Spark Job
- Generating Shakespeare Text with Spark and Long Short-Term Memory
- Setting Up the LSTM Network Architecture
- Training, Tracking Progress, and Understanding Results
- Modeling MNIST with a Convolutional Neural Network on Spark
- Configuring the Spark Job and Loading MNIST Data
- Setting Up the LeNet CNN Architecture and Training
- Introduction to Using DL4J with Spark and Hadoop
- A. What Is Artificial Intelligence?
- The Story So Far
- Defining Deep Learning
- Defining Artificial Intelligence
- The study of intelligence
- Cognitive dissonance and modern definitions
- What AI is not
- Moving the goal posts
- Segmenting the definitions of AI
- Critical commentary on segments
- A fifth aspirational definition of AI
- The AI winters
- AI Winter I: (19741980)
- AI Winter II: Late 1980s
- The common patterns of AI Winters
- What Is Driving Interest Today in AI Today?
- Winter Is Coming
- The Story So Far
- B. RL4J and Reinforcement Learning
- Preliminaries
- Markov Decision Process
- Terminology
- Different Settings
- Model-Free
- Observation Setting
- Single-Player and Adversarial Games
- Q-Learning
- From Policy to Neural Networks the following
- Policy Iteration
- Exploration Versus Exploitation
- Bellman Equation
- Initial State Sampling
- Q-Learning Implementation
- Modeling Q(s,a)
- Experience Replay
- Compression
- Convolutional Layers and Image Preprocessing
- Image processing
- History Processing
- Double Q-Learning
- Clipping
- Scaling Rewards
- Prioritized Replay
- Graph, Visualization, and Mean-Q
- RL4J
- Conclusion
- Preliminaries
- C. Numbers Everyone Should Know
- D. Neural Networks and Backpropagation: A Mathematical Approach
- Introduction
- Backpropagation in a Multilayer Perceptron
- E. Using the ND4J API
- Design and Basic Usage
- Understanding NDArrays
- ND4J General Syntax
- The Basics of Working with NDArrays
- The ND4J class
- Nd4j.zeros( int ... )
- Nd4j.ones( int ... )
- Initializing with other values
- Initializing with random numbers
- Controlling the shape of NDArrays
- Creating basic arrays
- Example: create a 2 x 2 NDArray
- Example: add two 2 x 2 NDArrays together
- Creating NDArrays from Java arrays
- Getting and setting individual NDArray values
- Working with NDArray rows
- Get a single row
- Get multiple rows
- Setting a single row
- Quick reference for determining the size/dimensions of NDArrays
- The ND4J class
- Dataset
- Relationship to NDArray
- Common uses
- Creating Input Vectors
- Basics of Vector Creation
- Sizing the vector
- Setting feature values
- Setting the label
- Single-label output
- Multiple-label output
- Regression output
- Basics of Vector Creation
- Using MLLibUtil
- Converting from INDArray to MLLib Vector
- Converting from MLLib Vector to INDArray
- Making Model Predictions with DL4J
- Using the DL4J and ND4J Together
- Differences between output vector depending on output layer type
- Logistic output layer for binary classification
- Softmax output layer for multilabel classification
- Linear output layer for regression output
- Getting the redicted label from the returned INDArray
- Differences between output vector depending on output layer type
- Using the DL4J and ND4J Together
- Design and Basic Usage
- F. Using DataVec
- Loading Data for Machine Learning
- Loading CSV Data for Multilayer Perceptrons
- Loading Image Data for Convolutional Neural Networks
- Loading Sequence Data for Recurrent Neural Networks
- Transforming Data: Data Wrangling with DataVec
- DataVec Transforms: Key Concepts
- DataVec Transform Functionality: An Example
- G. Working with DL4J from Source
- Verifying Git Is Installed
- Cloning Key DL4J GitHub Projects
- Downloading Source via Zip File
- Using Maven to Build Source Code
- H. Setting Up DL4J Projects
- Creating a New DL4J Project
- Java
- Working with Maven
- A minimal Project Object Model file
- Project Object Model explanation
- A minimal Project Object Model file
- IDEs
- Quickstart a DL4J project by using IntelliJ
- Setting Up Other Maven POMs
- ND4J and Maven
- Creating a New DL4J Project
- I. Setting Up GPUs for DL4J Projects
- Switching Backends to GPU
- Picking a GPU
- Training on a Multiple GPU System
- CUDA on Different Platforms
- Monitoring GPU Performance
- NVIDIA System Management Interface
- Switching Backends to GPU
- J. Troubleshooting DL4J Installations
- Previous Installation
- Memory Errors When Installing From Source
- Older Versions of Maven
- Maven and PATH Variables
- Bad JDK Versions
- C++ and Other Development Tools
- Windows and Include Paths
- Monitoring GPUs
- Using the JVisualVM
- Working with Clojure
- OS X and Float Support
- Fork-Join Bug in Java 7
- Precautions
- Other Local Repositories
- Check Maven Dependencies
- Reinstall Dependencies
- If All Else Fails
- Different Platforms
- OS X
- Windows
- Setting up Visual Studio
- Working with Windows on 64-bit platforms
- Linux
- Ubuntu
- Centos
- Index