Graph Algorithms. Practical Examples in Apache Spark and Neo4j - Helion
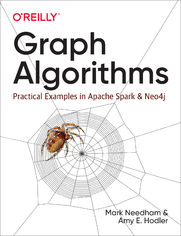
ISBN: 9781492047636
stron: 256, Format: ebook
Data wydania: 2019-05-16
Księgarnia: Helion
Cena książki: 211,65 zł (poprzednio: 246,10 zł)
Oszczędzasz: 14% (-34,45 zł)
Discover how graph algorithms can help you leverage the relationships within your data to develop more intelligent solutions and enhance your machine learning models. You’ll learn how graph analytics are uniquely suited to unfold complex structures and reveal difficult-to-find patterns lurking in your data. Whether you are trying to build dynamic network models or forecast real-world behavior, this book illustrates how graph algorithms deliver value—from finding vulnerabilities and bottlenecks to detecting communities and improving machine learning predictions.
This practical book walks you through hands-on examples of how to use graph algorithms in Apache Spark and Neo4j—two of the most common choices for graph analytics. Also included: sample code and tips for over 20 practical graph algorithms that cover optimal pathfinding, importance through centrality, and community detection.
- Learn how graph analytics vary from conventional statistical analysis
- Understand how classic graph algorithms work, and how they are applied
- Get guidance on which algorithms to use for different types of questions
- Explore algorithm examples with working code and sample datasets from Spark and Neo4j
- See how connected feature extraction can increase machine learning accuracy and precision
- Walk through creating an ML workflow for link prediction combining Neo4j and Spark
Osoby które kupowały "Graph Algorithms. Practical Examples in Apache Spark and Neo4j", wybierały także:
- Cisco CCNA 200-301. Kurs video. Administrowanie bezpieczeństwem sieci. Część 3 665,00 zł, (39,90 zł -94%)
- Cisco CCNA 200-301. Kurs video. Administrowanie urządzeniami Cisco. Część 2 665,00 zł, (39,90 zł -94%)
- Cisco CCNA 200-301. Kurs video. Podstawy sieci komputerowych i konfiguracji. Część 1 665,00 zł, (39,90 zł -94%)
- Cisco CCNP Enterprise 350-401 ENCOR. Kurs video. Programowanie i automatyzacja sieci 443,33 zł, (39,90 zł -91%)
- CCNP Enterprise 350-401 ENCOR. Kurs video. Mechanizmy kierowania ruchem pakiet 443,33 zł, (39,90 zł -91%)
Spis treści
Graph Algorithms. Practical Examples in Apache Spark and Neo4j eBook -- spis treści
- Preface
- Whats in This Book
- Conventions Used in This Book
- Using Code Examples
- OReilly Online Learning
- How to Contact Us
- Acknowledgments
- Foreword
- 1. Introduction
- What Are Graphs?
- What Are Graph Analytics and Algorithms?
- Graph Processing, Databases, Queries, and Algorithms
- OLTP and OLAP
- Why Should We Care About Graph Algorithms?
- Graph Analytics Use Cases
- Conclusion
- 2. Graph Theory and Concepts
- Terminology
- Graph Types and Structures
- Random, Small-World, Scale-Free Structures
- Flavors of Graphs
- Connected Versus Disconnected Graphs
- Unweighted Graphs Versus Weighted Graphs
- Undirected Graphs Versus Directed Graphs
- Acyclic Graphs Versus Cyclic Graphs
- Trees
- Sparse Graphs Versus Dense Graphs
- Monopartite, Bipartite, and k-Partite Graphs
- Types of Graph Algorithms
- Pathfinding
- Centrality
- Community Detection
- Summary
- 3. Graph Platforms and Processing
- Graph Platform and Processing Considerations
- Platform Considerations
- Processing Considerations
- Representative Platforms
- Selecting Our Platform
- Apache Spark
- Installing Spark
- Neo4j Graph Platform
- Installing Neo4j
- Summary
- Graph Platform and Processing Considerations
- 4. Pathfinding and Graph Search Algorithms
- Example Data: The Transport Graph
- Importing the Data into Apache Spark
- Importing the Data into Neo4j
- Breadth First Search
- Breadth First Search with Apache Spark
- Depth First Search
- Shortest Path
- When Should I Use Shortest Path?
- Shortest Path with Neo4j
- Shortest Path (Weighted) with Neo4j
- Shortest Path (Weighted) with Apache Spark
- Shortest Path Variation: A*
- A* with Neo4j
- Shortest Path Variation: Yens k-Shortest Paths
- Yens with Neo4j
- All Pairs Shortest Path
- A Closer Look at All Pairs Shortest Path
- When Should I Use All Pairs Shortest Path?
- All Pairs Shortest Path with Apache Spark
- All Pairs Shortest Path with Neo4j
- Single Source Shortest Path
- When Should I Use Single Source Shortest Path?
- Single Source Shortest Path with Apache Spark
- Single Source Shortest Path with Neo4j
- Minimum Spanning Tree
- When Should I Use Minimum Spanning Tree?
- Minimum Spanning Tree with Neo4j
- Random Walk
- When Should I Use Random Walk?
- Random Walk with Neo4j
- Summary
- Example Data: The Transport Graph
- 5. Centrality Algorithms
- Example Graph Data: The Social Graph
- Importing the Data into Apache Spark
- Importing the Data into Neo4j
- Degree Centrality
- Reach
- When Should I Use Degree Centrality?
- Degree Centrality with Apache Spark
- Closeness Centrality
- When Should I Use Closeness Centrality?
- Closeness Centrality with Apache Spark
- Closeness Centrality with Neo4j
- Closeness Centrality Variation: Wasserman and Faust
- Closeness Centrality Variation: Harmonic Centrality
- Harmonic Centrality with Neo4j
- Betweenness Centrality
- Bridges and control points
- Calculating betweenness centrality
- When Should I Use Betweenness Centrality?
- Betweenness Centrality with Neo4j
- Betweenness Centrality Variation: Randomized-Approximate Brandes
- Random
- Degree
- Approximation of Betweenness Centrality with Neo4j
- PageRank
- Influence
- The PageRank Formula
- Iteration, Random Surfers, and Rank Sinks
- When Should I Use PageRank?
- PageRank with Apache Spark
- PageRank with a fixed number of iterations
- PageRank until convergence
- PageRank with Neo4j
- PageRank Variation: Personalized PageRank
- Personalized PageRank with Apache Spark
- Summary
- Example Graph Data: The Social Graph
- 6. Community Detection Algorithms
- Example Graph Data: The Software Dependency Graph
- Importing the Data into Apache Spark
- Importing the Data into Neo4j
- Triangle Count and Clustering Coefficient
- Local Clustering Coefficient
- Global Clustering Coefficient
- When Should I Use Triangle Count and Clustering Coefficient?
- Triangle Count with Apache Spark
- Triangles with Neo4j
- Local Clustering Coefficient with Neo4j
- Strongly Connected Components
- When Should I Use Strongly Connected Components?
- Strongly Connected Components with Apache Spark
- Strongly Connected Components with Neo4j
- Connected Components
- When Should I Use Connected Components?
- Connected Components with Apache Spark
- Connected Components with Neo4j
- Label Propagation
- Semi-Supervised Learning and Seed Labels
- When Should I Use Label Propagation?
- Label Propagation with Apache Spark
- Label Propagation with Neo4j
- Louvain Modularity
- Quality-based grouping via modularity
- When Should I Use Louvain?
- Louvain with Neo4j
- Validating Communities
- Summary
- Example Graph Data: The Software Dependency Graph
- 7. Graph Algorithms in Practice
- Analyzing Yelp Data with Neo4j
- Yelp Social Network
- Data Import
- Graph Model
- A Quick Overview of the Yelp Data
- Trip Planning App
- Finding influential hotel reviewers
- Travel Business Consulting
- Bellagio cross-promotion
- Finding Similar Categories
- Analyzing Airline Flight Data with Apache Spark
- Exploratory Analysis
- Popular Airports
- Delays from ORD
- Bad Day at SFO
- Interconnected Airports by Airline
- Summary
- Analyzing Yelp Data with Neo4j
- 8. Using Graph Algorithms to Enhance Machine Learning
- Machine Learning and the Importance of Context
- Graphs, Context, and Accuracy
- Connected Feature Extraction and Selection
- Graphy Features
- Graph Algorithm Features
- Graphs and Machine Learning in Practice: Link Prediction
- Tools and Data
- Importing the Data into Neo4j
- The Coauthorship Graph
- Creating Balanced Training and Testing Datasets
- Balancing and splitting data
- How We Predict Missing Links
- Creating a Machine Learning Pipeline
- Predicting Links: Basic Graph Features
- Predicting Links: Triangles and the Clustering Coefficient
- Predicting Links: Community Detection
- Summary
- Wrapping Things Up
- Machine Learning and the Importance of Context
- A. Additional Information and Resources
- Other Algorithms
- Neo4j Bulk Data Import and Yelp
- APOC and Other Neo4j Tools
- Finding Datasets
- Assistance with the Apache Spark and Neo4j Platforms
- Training
- Index