Designing Machine Learning Systems - Helion
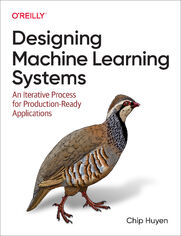
ISBN: 9781098107918
stron: 388, Format: ebook
Data wydania: 2022-05-17
Księgarnia: Helion
Cena książki: 229,00 zł
Machine learning systems are both complex and unique. Complex because they consist of many different components and involve many different stakeholders. Unique because they're data dependent, with data varying wildly from one use case to the next. In this book, you'll learn a holistic approach to designing ML systems that are reliable, scalable, maintainable, and adaptive to changing environments and business requirements.
Author Chip Huyen, co-founder of Claypot AI, considers each design decision--such as how to process and create training data, which features to use, how often to retrain models, and what to monitor--in the context of how it can help your system as a whole achieve its objectives. The iterative framework in this book uses actual case studies backed by ample references.
This book will help you tackle scenarios such as:
- Engineering data and choosing the right metrics to solve a business problem
- Automating the process for continually developing, evaluating, deploying, and updating models
- Developing a monitoring system to quickly detect and address issues your models might encounter in production
- Architecting an ML platform that serves across use cases
- Developing responsible ML systems
Osoby które kupowały "Designing Machine Learning Systems", wybierały także:
- PLC i TIA Portal. Kurs video. Techniki programowania SIMATIC S7-1200 200,00 zł, (10,00 zł -95%)
- Google Cloud. Kurs video. Zostań administratorem systemów IT 142,86 zł, (10,00 zł -93%)
- Instalacja i konfiguracja baz danych. Kurs video. Przygotowanie do egzaminu 70-765 Provisioning SQL Databases 285,57 zł, (19,99 zł -93%)
- Jak zhakowa 125,00 zł, (10,00 zł -92%)
- Excel w HR. Kurs video. Od teorii do praktyki 222,11 zł, (19,99 zł -91%)
Spis treści
Designing Machine Learning Systems eBook -- spis treści
- Preface
- Who This Book Is For
- What This Book Is Not
- Navigating This Book
- GitHub Repository and Community
- Conventions Used in This Book
- Using Code Examples
- OReilly Online Learning
- How to Contact Us
- Acknowledgments
- 1. Overview of Machine Learning Systems
- When to Use Machine Learning
- Machine Learning Use Cases
- Understanding Machine Learning Systems
- Machine Learning in Research Versus in Production
- Different stakeholders and requirements
- Computational priorities
- Data
- Fairness
- Interpretability
- Discussion
- Machine Learning Systems Versus Traditional Software
- Machine Learning in Research Versus in Production
- Summary
- When to Use Machine Learning
- 2. Introduction to Machine Learning Systems Design
- Business and ML Objectives
- Requirements for ML Systems
- Reliability
- Scalability
- Maintainability
- Adaptability
- Iterative Process
- Framing ML Problems
- Types of ML Tasks
- Classification versus regression
- Binary versus multiclass classification
- Multiclass versus multilabel classification
- Multiple ways to frame a problem
- Objective Functions
- Decoupling objectives
- Types of ML Tasks
- Mind Versus Data
- Summary
- 3. Data Engineering Fundamentals
- Data Sources
- Data Formats
- JSON
- Row-Major Versus Column-Major Format
- Text Versus Binary Format
- Data Models
- Relational Model
- NoSQL
- Document model
- Graph model
- Structured Versus Unstructured Data
- Data Storage Engines and Processing
- Transactional and Analytical Processing
- ETL: Extract, Transform, and Load
- Modes of Dataflow
- Data Passing Through Databases
- Data Passing Through Services
- Data Passing Through Real-Time Transport
- Batch Processing Versus Stream Processing
- Summary
- 4. Training Data
- Sampling
- Nonprobability Sampling
- Simple Random Sampling
- Stratified Sampling
- Weighted Sampling
- Reservoir Sampling
- Importance Sampling
- Labeling
- Hand Labels
- Label multiplicity
- Data lineage
- Natural Labels
- Feedback loop length
- Handling the Lack of Labels
- Weak supervision
- Semi-supervision
- Transfer learning
- Active learning
- Hand Labels
- Class Imbalance
- Challenges of Class Imbalance
- Handling Class Imbalance
- Using the right evaluation metrics
- Data-level methods: Resampling
- Algorithm-level methods
- Cost-sensitive learning
- Class-balanced loss
- Focal loss
- Data Augmentation
- Simple Label-Preserving Transformations
- Perturbation
- Data Synthesis
- Summary
- Sampling
- 5. Feature Engineering
- Learned Features Versus Engineered Features
- Common Feature Engineering Operations
- Handling Missing Values
- Deletion
- Imputation
- Scaling
- Discretization
- Encoding Categorical Features
- Feature Crossing
- Discrete and Continuous Positional Embeddings
- Handling Missing Values
- Data Leakage
- Common Causes for Data Leakage
- Splitting time-correlated data randomly instead of by time
- Scaling before splitting
- Filling in missing data with statistics from the test split
- Poor handling of data duplication before splitting
- Group leakage
- Leakage from data generation process
- Detecting Data Leakage
- Common Causes for Data Leakage
- Engineering Good Features
- Feature Importance
- Feature Generalization
- Summary
- 6. Model Development and Offline Evaluation
- Model Development and Training
- Evaluating ML Models
- Six tips for model selection
- Avoid the state-of-the-art trap
- Start with the simplest models
- Avoid human biases in selecting models
- Evaluate good performance now versus good performance later
- Evaluate trade-offs
- Understand your models assumptions
- Six tips for model selection
- Ensembles
- Bagging
- Boosting
- Stacking
- Experiment Tracking and Versioning
- Experiment tracking
- Versioning
- Distributed Training
- Data parallelism
- Model parallelism
- AutoML
- Soft AutoML: Hyperparameter tuning
- Hard AutoML: Architecture search and learned optimizer
- Evaluating ML Models
- Model Offline Evaluation
- Baselines
- Evaluation Methods
- Perturbation tests
- Invariance tests
- Directional expectation tests
- Model calibration
- Confidence measurement
- Slice-based evaluation
- Summary
- Model Development and Training
- 7. Model Deployment and Prediction Service
- Machine Learning Deployment Myths
- Myth 1: You Only Deploy One or Two ML Models at a Time
- Myth 2: If We Dont Do Anything, Model Performance Remains the Same
- Myth 3: You Wont Need to Update Your Models as Much
- Myth 4: Most ML Engineers Dont Need to Worry About Scale
- Batch Prediction Versus Online Prediction
- From Batch Prediction to Online Prediction
- Unifying Batch Pipeline and Streaming Pipeline
- Model Compression
- Low-Rank Factorization
- Knowledge Distillation
- Pruning
- Quantization
- ML on the Cloud and on the Edge
- Compiling and Optimizing Models for Edge Devices
- Model optimization
- Using ML to optimize ML models
- ML in Browsers
- Compiling and Optimizing Models for Edge Devices
- Summary
- Machine Learning Deployment Myths
- 8. Data Distribution Shifts and Monitoring
- Causes of ML System Failures
- Software System Failures
- ML-Specific Failures
- Production data differing from training data
- Edge cases
- Degenerate feedback loops
- Detecting degenerate feedback loops
- Correcting degenerate feedback loops
- Data Distribution Shifts
- Types of Data Distribution Shifts
- Covariate shift
- Label shift
- Concept drift
- General Data Distribution Shifts
- Detecting Data Distribution Shifts
- Statistical methods
- Time scale windows for detecting shifts
- Addressing Data Distribution Shifts
- Types of Data Distribution Shifts
- Monitoring and Observability
- ML-Specific Metrics
- Monitoring accuracy-related metrics
- Monitoring predictions
- Monitoring features
- Monitoring raw inputs
- Monitoring Toolbox
- Logs
- Dashboards
- Alerts
- Observability
- ML-Specific Metrics
- Summary
- Causes of ML System Failures
- 9. Continual Learning and Test in Production
- Continual Learning
- Stateless Retraining Versus Stateful Training
- Why Continual Learning?
- Continual Learning Challenges
- Fresh data access challenge
- Evaluation challenge
- Algorithm challenge
- Four Stages of Continual Learning
- Stage 1: Manual, stateless retraining
- Stage 2: Automated retraining
- Requirements
- Stage 3: Automated, stateful training
- Requirements
- Stage 4: Continual learning
- Requirements
- How Often to Update Your Models
- Value of data freshness
- Model iteration versus data iteration
- Test in Production
- Shadow Deployment
- A/B Testing
- Canary Release
- Interleaving Experiments
- Bandits
- Contextual bandits as an exploration strategy
- Summary
- Continual Learning
- 10. Infrastructure and Tooling for MLOps
- Storage and Compute
- Public Cloud Versus Private Data Centers
- Development Environment
- Dev Environment Setup
- IDE
- Standardizing Dev Environments
- From Dev to Prod: Containers
- Dev Environment Setup
- Resource Management
- Cron, Schedulers, and Orchestrators
- Data Science Workflow Management
- ML Platform
- Model Deployment
- Model Store
- Feature Store
- Build Versus Buy
- Summary
- Storage and Compute
- 11. The Human Side of Machine Learning
- User Experience
- Ensuring User Experience Consistency
- Combatting Mostly Correct Predictions
- Smooth Failing
- Team Structure
- Cross-functional Teams Collaboration
- End-to-End Data Scientists
- Approach 1: Have a separate team to manage production
- Approach 2: Data scientists own the entire process
- Responsible AI
- Irresponsible AI: Case Studies
- Case study I: Automated graders biases
- Failure 1: Setting the wrong objective
- Failure 2: Insufficient fine-grained model evaluation to discover biases
- Failure 3: Lack of transparency
- Case study II: The danger of anonymized data
- Case study I: Automated graders biases
- A Framework for Responsible AI
- Discover sources for model biases
- Understand the limitations of the data-driven approach
- Understand the trade-offs between different desiderata
- Act early
- Create model cards
- Establish processes for mitigating biases
- Stay up-to-date on responsible AI
- Irresponsible AI: Case Studies
- Summary
- User Experience
- Epilogue
- Index