Programming Computer Vision with Python. Tools and algorithms for analyzing images - Helion
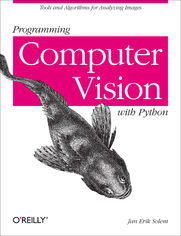
ISBN: 978-14-493-4193-0
stron: 264, Format: ebook
Data wydania: 2012-06-19
Księgarnia: Helion
Cena książki: 186,15 zł (poprzednio: 216,45 zł)
Oszczędzasz: 14% (-30,30 zł)
If you want a basic understanding of computer vision’s underlying theory and algorithms, this hands-on introduction is the ideal place to start. You’ll learn techniques for object recognition, 3D reconstruction, stereo imaging, augmented reality, and other computer vision applications as you follow clear examples written in Python.
Programming Computer Vision with Python explains computer vision in broad terms that won’t bog you down in theory. You get complete code samples with explanations on how to reproduce and build upon each example, along with exercises to help you apply what you’ve learned. This book is ideal for students, researchers, and enthusiasts with basic programming and standard mathematical skills.
- Learn techniques used in robot navigation, medical image analysis, and other computer vision applications
- Work with image mappings and transforms, such as texture warping and panorama creation
- Compute 3D reconstructions from several images of the same scene
- Organize images based on similarity or content, using clustering methods
- Build efficient image retrieval techniques to search for images based on visual content
- Use algorithms to classify image content and recognize objects
- Access the popular OpenCV library through a Python interface
Osoby które kupowały "Programming Computer Vision with Python. Tools and algorithms for analyzing images", wybierały także:
- Algorytmy kryptograficzne. Przewodnik po algorytmach w blockchain, kryptografii kwantowej, protoko 79,00 zł, (39,50 zł -50%)
- Informatyk samouk. Przewodnik po strukturach danych i algorytmach dla pocz 58,98 zł, (29,49 zł -50%)
- My 89,00 zł, (44,50 zł -50%)
- Nauka algorytm 58,98 zł, (29,49 zł -50%)
- 40 algorytmów, które powinien znać każdy programista. Nauka implementacji algorytmów w Pythonie 77,00 zł, (38,50 zł -50%)
Spis treści
Programming Computer Vision with Python. Tools and algorithms for analyzing images eBook -- spis treści
- Programming Computer Vision with Python
- SPECIAL OFFER: Upgrade this ebook with OReilly
- Preface
- Prerequisites and Overview
- What You Need to Know
- What You Will Learn
- Chapter Overview
- Introduction to Computer Vision
- Python and NumPy
- Notation and Conventions
- Using Code Examples
- How to Contact Us
- Safari Books Online
- Acknowledgments
- Prerequisites and Overview
- 1. Basic Image Handling and Processing
- 1.1 PILThe Python Imaging Library
- Convert Images to Another Format
- Create Thumbnails
- Copy and Paste Regions
- Resize and Rotate
- 1.2 Matplotlib
- Plotting Images, Points, and Lines
- Image Contours and Histograms
- Interactive Annotation
- 1.3 NumPy
- Array Image Representation
- Graylevel Transforms
- Image Resizing
- Histogram Equalization
- Averaging Images
- PCA of Images
- Using the Pickle Module
- 1.4 SciPy
- Blurring Images
- Image Derivatives
- MorphologyCounting Objects
- Useful SciPy Modules
- Reading and writing .mat files
- Saving arrays as images
- 1.5 Advanced Example: Image De-Noising
- Exercises
- Conventions for the Code Examples
- 1.1 PILThe Python Imaging Library
- 2. Local Image Descriptors
- 2.1 Harris Corner Detector
- Finding Corresponding Points Between Images
- 2.2 SIFTScale-Invariant Feature Transform
- Interest Points
- Descriptor
- Detecting Interest Points
- Matching Descriptors
- 2.3 Matching Geotagged Images
- Downloading Geotagged Images from Panoramio
- Matching Using Local Descriptors
- Visualizing Connected Images
- Exercises
- 2.1 Harris Corner Detector
- 3. Image to Image Mappings
- 3.1 Homographies
- The Direct Linear Transformation Algorithm
- Affine Transformations
- 3.2 Warping Images
- Image in Image
- Piecewise Affine Warping
- Registering Images
- 3.3 Creating Panoramas
- RANSAC
- Robust Homography Estimation
- Stitching the Images Together
- Exercises
- 3.1 Homographies
- 4. Camera Models and Augmented Reality
- 4.1 The Pin-Hole Camera Model
- The Camera Matrix
- Projecting 3D Points
- Factoring the Camera Matrix
- Computing the Camera Center
- 4.2 Camera Calibration
- A Simple Calibration Method
- 4.3 Pose Estimation from Planes and Markers
- 4.4 Augmented Reality
- PyGame and PyOpenGL
- From Camera Matrix to OpenGL Format
- Placing Virtual Objects in the Image
- Tying It All Together
- Loading Models
- Exercises
- 4.1 The Pin-Hole Camera Model
- 5. Multiple View Geometry
- 5.1 Epipolar Geometry
- A Sample Data Set
- Plotting 3D Data with Matplotlib
- Computing FThe Eight Point Algorithm
- The Epipole and Epipolar Lines
- 5.2 Computing with Cameras and 3D Structure
- Triangulation
- Computing the Camera Matrix from 3D Points
- Computing the Camera Matrix from a Fundamental Matrix
- The uncalibrated caseprojective reconstruction
- The calibrated casemetric reconstruction
- 5.3 Multiple View Reconstruction
- Robust Fundamental Matrix Estimation
- 3D Reconstruction Example
- Extensions and More Than Two Views
- More views
- Bundle adjustment
- Self-calibration
- 5.4 Stereo Images
- Computing Disparity Maps
- Exercises
- 5.1 Epipolar Geometry
- 6. Clustering Images
- 6.1 K-Means Clustering
- The SciPy Clustering Package
- Clustering Images
- Visualizing the Images on Principal Components
- Clustering Pixels
- 6.2 Hierarchical Clustering
- Clustering Images
- 6.3 Spectral Clustering
- Exercises
- 6.1 K-Means Clustering
- 7. Searching Images
- 7.1 Content-Based Image Retrieval
- Inspiration from Text MiningThe Vector Space Model
- 7.2 Visual Words
- Creating a Vocabulary
- 7.3 Indexing Images
- Setting Up the Database
- Adding Images
- 7.4 Searching the Database for Images
- Using the Index to Get Candidates
- Querying with an Image
- Benchmarking and Plotting the Results
- 7.5 Ranking Results Using Geometry
- 7.6 Building Demos and Web Applications
- Creating Web Applications with CherryPy
- Image Search Demo
- Exercises
- 7.1 Content-Based Image Retrieval
- 8. Classifying Image Content
- 8.1 K-Nearest Neighbors
- A Simple 2D Example
- Dense SIFT as Image Feature
- Classifying ImagesHand Gesture Recognition
- 8.2 Bayes Classifier
- Using PCA to Reduce Dimensions
- 8.3 Support Vector Machines
- Using LibSVM
- Hand Gesture Recognition Again
- 8.4 Optical Character Recognition
- Training a Classifier
- Selecting Features
- Multi-Class SVM
- Extracting Cells and Recognizing Characters
- Rectifying Images
- Exercises
- 8.1 K-Nearest Neighbors
- 9. Image Segmentation
- 9.1 Graph Cuts
- Graphs from Images
- Segmentation with User Input
- 9.2 Segmentation Using Clustering
- 9.3 Variational Methods
- Exercises
- 9.1 Graph Cuts
- 10. OpenCV
- 10.1 The OpenCV Python Interface
- 10.2 OpenCV Basics
- Reading and Writing Images
- Color Spaces
- Displaying Images and Results
- 10.3 Processing Video
- Video Input
- Reading Video to NumPy Arrays
- 10.4 Tracking
- Optical Flow
- The Lucas-Kanade Algorithm
- Using the tracker
- Using generators
- 10.5 More Examples
- Inpainting
- Segmentation with the Watershed Transform
- Line Detection with a Hough Transform
- Exercises
- A. Installing Packages
- A.1 NumPy and SciPy
- Windows
- Mac OS X
- Linux
- A.2 Matplotlib
- A.3 PIL
- A.4 LibSVM
- A.5 OpenCV
- Windows and Unix
- Mac OS X
- Linux
- A.6 VLFeat
- A.7 PyGame
- A.8 PyOpenGL
- A.9 Pydot
- A.10 Python-graph
- A.11 Simplejson
- A.12 PySQLite
- A.13 CherryPy
- A.1 NumPy and SciPy
- B. Image Datasets
- B.1 Flickr
- B.2 Panoramio
- B.3 Oxford Visual Geometry Group
- B.4 University of Kentucky Recognition Benchmark Images
- B.5 Other
- Prague Texture Segmentation Datagenerator and Benchmark
- MSR Cambridge Grab Cut Dataset
- Caltech 101
- Static Hand Posture Database
- Middlebury Stereo Datasets
- C. Image Credits
- C.1 Images from Flickr
- C.2 Other Images
- C.3 Illustrations
- D. References
- E. About the Author
- Index
- About the Author
- Colophon
- SPECIAL OFFER: Upgrade this ebook with OReilly
- Copyright