Essential PySpark for Scalable Data Analytics - Helion
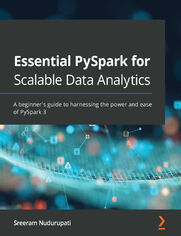
Tytuł oryginału: Essential PySpark for Scalable Data Analytics
ISBN: 9781800563094
stron: 322, Format: ebook
Data wydania: 2021-10-29
Księgarnia: Helion
Cena książki: 139,00 zł
Get started with distributed computing using PySpark, a single unified framework to solve end-to-end data analytics at scale
Key Features
- Discover how to convert huge amounts of raw data into meaningful and actionable insights
- Use Spark's unified analytics engine for end-to-end analytics, from data preparation to predictive analytics
- Perform data ingestion, cleansing, and integration for ML, data analytics, and data visualization
Book Description
Apache Spark is a unified data analytics engine designed to process huge volumes of data quickly and efficiently. PySpark is Apache Spark's Python language API, which offers Python developers an easy-to-use scalable data analytics framework.
Essential PySpark for Scalable Data Analytics starts by exploring the distributed computing paradigm and provides a high-level overview of Apache Spark. You'll begin your analytics journey with the data engineering process, learning how to perform data ingestion, cleansing, and integration at scale. This book helps you build real-time analytics pipelines that help you gain insights faster. You'll then discover methods for building cloud-based data lakes, and explore Delta Lake, which brings reliability to data lakes. The book also covers Data Lakehouse, an emerging paradigm, which combines the structure and performance of a data warehouse with the scalability of cloud-based data lakes. Later, you'll perform scalable data science and machine learning tasks using PySpark, such as data preparation, feature engineering, and model training and productionization. Finally, you'll learn ways to scale out standard Python ML libraries along with a new pandas API on top of PySpark called Koalas.
By the end of this PySpark book, you'll be able to harness the power of PySpark to solve business problems.
What you will learn
- Understand the role of distributed computing in the world of big data
- Gain an appreciation for Apache Spark as the de facto go-to for big data processing
- Scale out your data analytics process using Apache Spark
- Build data pipelines using data lakes, and perform data visualization with PySpark and Spark SQL
- Leverage the cloud to build truly scalable and real-time data analytics applications
- Explore the applications of data science and scalable machine learning with PySpark
- Integrate your clean and curated data with BI and SQL analysis tools
Who this book is for
This book is for practicing data engineers, data scientists, data analysts, and data enthusiasts who are already using data analytics to explore distributed and scalable data analytics. Basic to intermediate knowledge of the disciplines of data engineering, data science, and SQL analytics is expected. General proficiency in using any programming language, especially Python, and working knowledge of performing data analytics using frameworks such as pandas and SQL will help you to get the most out of this book.
Osoby które kupowały "Essential PySpark for Scalable Data Analytics", wybierały także:
- Data Science w Pythonie. Kurs video. Przetwarzanie i analiza danych 149,00 zł, (67,05 zł -55%)
- Excel 2013. Kurs video. Poziom drugi. Przetwarzanie i analiza danych 79,00 zł, (35,55 zł -55%)
- Power Automate. Kurs video. Automatyzacja proces 109,00 zł, (54,50 zł -50%)
- Power Apps. Kurs video. Tworzenie biznesowych aplikacji no-code 198,98 zł, (99,49 zł -50%)
- Data science od podstaw. Analiza danych w Pythonie. Wydanie II 67,00 zł, (33,50 zł -50%)
Spis treści
Essential PySpark for Scalable Data Analytics. A beginner's guide to harnessing the power and ease of PySpark 3 eBook -- spis treści
- 1. Distributed Computing Primer
- 2. Data Ingestion
- 3. Data Cleansing and Integration
- 4. Real-time Data Analytics
- 5. Scalable Machine Learning with PySpark
- 6. Feature Engineering – Extraction, Transformation, and Selection
- 7. Supervised Machine Learning
- 8. Unsupervised Machine Learning
- 9. Machine Learning Life Cycle Management
- 10. Scaling Out Single-Node Machine Learning Using PySpark
- 11. Data Visualization with PySpark
- 12. Spark SQL Primer
- 13. Integrating External Tools with Spark SQL
- 14. The Data Lakehouse